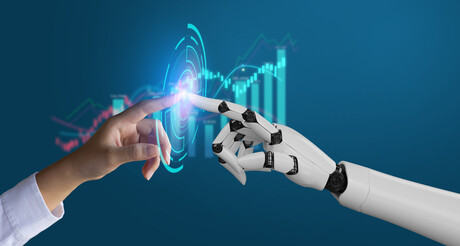
The so-called ‘reproducibility crisis’ is among the most significant challenges facing scientists across all disciplines.
Ultimately, the nature of experiment replication is time-consuming and expensive. Combining those factors with a toxic ‘publish or perish’ culture in research (and a heavy bias towards positive results in scientific publishing) creates the perfect storm that has plagued the community for decades.
There’s no perfect solution to this issue, but one way researchers may be able to address the reproducibility crisis in the future is with artificial intelligence (AI) to develop life science experiments in a cloud laboratory environment. A host of prominent thinkers in AI have recently joined an AI Scientific Advisory Board to tackle the tough topics, from ethics to experimentation.
What’s behind the reproducibility crisis?
When we say ‘reproducibility crisis’, we’re specifically talking about an inability to demonstrate consistent results after using an established experimental methodology. A study in Nature of over 1500 researchers showed that more than 70% were unable to reproduce another scientist’s experiments. Many of those surveyed weren’t even able to replicate their own experiments.
What is contributing to this reproducibility crisis? In some cases, it is the pressure felt by researchers to publish findings in academic journals that often are heavily biased towards statistically significant results. That leads to instances of selective reporting and cherry-picking data results, which in turn leads to irreproducible research.
This is, unfortunately, a consequence of the ‘publish or perish’ mindset in academia. This part of the reproducibility problem is unlikely to be resolved unless the academic scientific community revises its thinking about how researchers are evaluated. The other side of the problem demands better detailed experimental methodology, along with comprehensive data capture of every aspect of the experiment from reagent to sample preparation to results. That part of the problem can and should be addressed by the industry, combining new scientific technology with innovations in artificial intelligence.
A well-funded but still underappreciated category of laboratory technology is becoming known generically as the cloud lab. Offering multiple categories of scientific equipment to run experiments using the internet as the backbone, cloud labs can address the reproducibility crisis by helping scientists incorporate more precise details into experiment methodology and comprehensive metadata.
Cloud labs are secure and highly automated, with experimental methods that are annotated with complete metadata. This leads to a more comprehensive understanding of potentially variable factors ranging from ambient temperature to instrument settings and configuration. That by itself can help shed light on reproducibility problems.
Further, cloud labs offer round-the-clock access to lab equipment, with processes running in the background while other research is conducted. In a cloud lab, science never sleeps. That means cloud labs can reduce the time required to replicate studies from months to weeks.
Cloud labs also help control the cost of experimentation. A subscription fee for access to the comprehensive capabilities of a cloud lab is often less than the cost a research organisation might otherwise pay for a single category of a lab instrument.
Learning to use cloud lab technology and coding experiments in that environment can be a daunting task for some researchers, however. That’s why the industry is starting to move towards incorporating AI both to create experiments and to smooth the transition to cloud lab experimentation.
Some industry observers believe AI and automated cloud labs have the potential to be even better than human scientists. The argument is that AI can, to all intents and purposes, read every protocol ever written and process metadata regarding every experiment ever executed within a cloud lab.
Walking before we run: scientists weigh in on ethical AI
That’s not to say that AI should immediately be integrated into all aspects of life sciences experimentation. As the saying goes, you have to walk before you can run, to make sure perceived ethical problems are adequately addressed. By doing so, the industry as a whole can avoid what Wired magazine called “sloppy use of machine learning” in science, where researchers have rushed to use machine learning, only to inadvertently introduce errors into findings.
To take a more measured and responsible approach to the introduction of AI into life sciences experimentation, a new AI Scientific Advisory Board, spearheaded by Emerald Cloud Lab, launched in April. The purpose of the board is to weigh in on both the ethical and methodological implications of AI both in the cloud lab environment and beyond.
The AI Scientific Advisory Board comprises some of the leading experts in AI and scientific experimentation:
- Erika Alden DeBenedictis, PhD: Computational physicist and synthetic biologist at the Francis Crick Institute
- Dr Andrew Trister, MD Medicine, PhD in Bioengineering: Deputy Director of Digital Health and AI at the Bill and Melinda Gates Foundation
- Gabe Gomes, PhD: Assistant Professor at Carnegie Mellon University
- Stephen Wolfram, PhD: Founder and CEO of Wolfram Research and Wolfram Alpha
- Christopher Wolfram, Software Engineer at Wolfram
- Armaghan Naik, PhD: CEO of Avronna
Working cooperatively, the board’s mission is to propose new guidelines and ethical frameworks for AI in the laboratory environment. In addition to potentially accelerating scientific progress and democratising access to scientific resources, the board sees AI as an important new means of enhancing interdisciplinary collaboration.
AI offers many potential advantages, including making it easier to design experiments and analyse data. Nonetheless, there is a need for appropriate policies, procedures and methods — and a thorough consideration of ethical standards — before AI functionality can be responsibly integrated into a highly automated lab environment. Ultimately, however, AI-driven research will be able to provide researchers with access to quality structured data necessary for both simulated and real-life experimentation.
And with that, the problem of the reproducibility crises in life sciences will be a step closer to being answered.